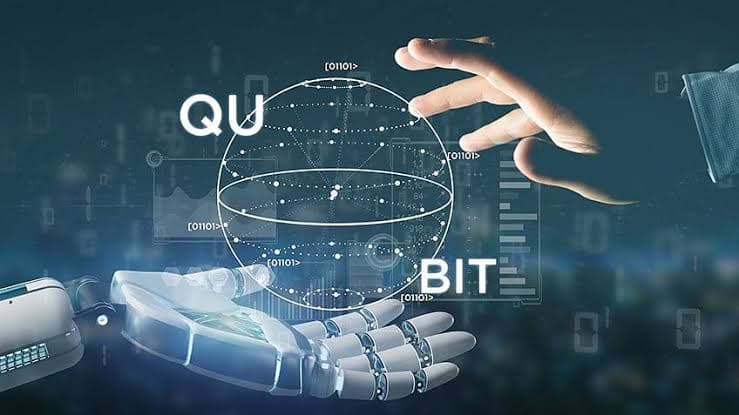
Quantum Machine Learning : The Future Of Artificial Intelligence.
July 20, 2023
This blog post discusses the latest trend in artificial intelligence and machine learning, known as quantum machine learning. It explores what quantum machine learning is, its potential applications, and how it differs from classical machine learning. The post also highlights the impact it may have on various industries and the challenges faced in its implementation. The article concludes by emphasizing quantum machine learning as a promising technology that has the potential to revolutionize the field of artificial intelligence and machine learning.
AI And ML
Robotics
Quantum Machine Learning: The Future of Artificial Intelligence
Quantum machine learning is the latest trend in the field of artificial intelligence and machine learning. It is a novel approach to machine learning that has the potential to revolutionize the industry and pave the way for new discoveries and breakthroughs.
In this post, we will explore what is quantum machine learning, its applications, and how it differs from classical machine learning. We will also discuss the impact it may have on various industries and the challenges that still exist in its implementation.
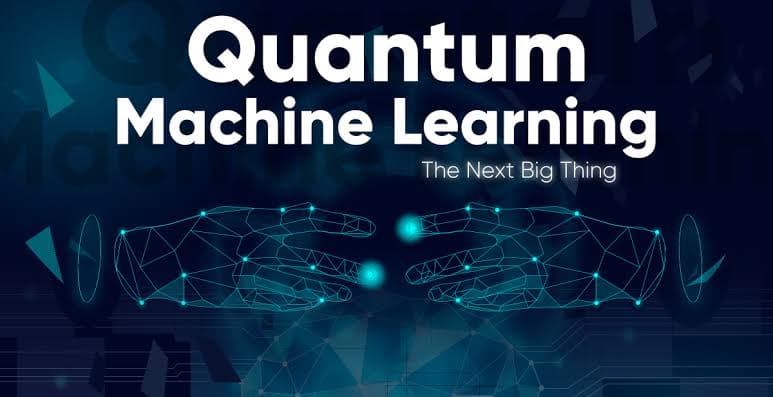
What is Quantum Machine Learning?
Quantum machine learning is the integration of quantum computing and machine learning. Quantum computing has the potential to solve problems that are intractable for classical computers. This includes tasks such as optimizing complex systems, simulating quantum systems, and solving mathematical problems.
Machine learning, on the other hand, is a technique that enables a computer system to learn from data and improve its performance over time. It is widely used in various industries, including finance, healthcare, and e-commerce, to make predictions, detect patterns, and analyze data.
Quantum machine learning combines the power of quantum computing with machine learning to create new algorithms that can process and analyze data more efficiently.
Applications of Quantum Machine Learning
Quantum machine learning has many potential applications in various fields, including finance, healthcare, transportation, and more. Here are some examples of how quantum machine learning can be used:
- Drug discovery: Quantum machine learning can help identify new drugs more efficiently, which can save time and money for pharmaceutical companies.
- Fraud detection: Quantum machine learning algorithms can detect fraud patterns more accurately than classical methods, which can help prevent financial loss for companies.
- Weather forecasting: Quantum machine learning algorithms can analyze large amounts of weather data and provide more accurate forecasting, which can help save lives during natural disasters.
How is Quantum Machine Learning Different from Classical Machine Learning?
Quantum machine learning differs from classical machine learning in several ways. Here are some of the key differences:
- Quantum machine learning algorithms use qubits instead of classical bits.
- Quantum machine learning algorithms can perform multiple calculations simultaneously, making them more efficient than classical algorithms.
- Quantum machine learning algorithms can process and analyze data that is encrypted, which can be beneficial for cybersecurity.
Challenges in Implementing Quantum Machine Learning
Despite its potential, there are still some challenges in implementing quantum machine learning. Here are some of the key challenges:
- Lack of expertise: There is a shortage of experts in both quantum computing and machine learning, which can pose a challenge for companies looking to implement quantum machine learning.
- Complexity: Quantum machine learning algorithms are complex and require advanced mathematical knowledge.
- Cost: Quantum computers are expensive, and not all companies can afford them.
Conclusion
Quantum machine learning is a promising technology that has the potential to revolutionize the field of artificial intelligence and machine learning. It has many potential applications and is already being used in various industries. However, challenges remain in its implementation, and further research is needed to fully realize its potential.
See moreLeave a Reply
Your email address will not be published.
Required fields are marked*
Comment *
Name*
Email*